Credit Risk Analysis Models
These are the techniques banks and creditors use to assess the degree of credit risk they bear while extending loans.
What are Credit Risk Analysis Models?
The Credit Risk Analysis Models are the techniques banks and creditors use to assess the degree of credit risk they bear while extending loans.
Credit Risk Analysis Models are used for debt contracts that legally bind a debtor and a creditor to satisfy their terms and pay off the debt obligations.
In this case, a debtor is a party that borrows the money and is imposed to repay the principal amount of the loan, along with interest. In comparison, a creditor is the party that extends the loan, which is usually the bank or a financial institution.
The interest levied on the debt is viewed as the creditor's reward for extending the debt and bearing the credit risk. The borrower may not pay the loan amount and interest to the bank, which creates a risk related to the probability of default, known as credit risk.
Banks are advised to implement measures that minimize the probability of defaults to mitigate credit risk, even though an accurate evaluation and precise determination of credit risk likelihood may be challenging.
In light of this, banks analyze credit risk using models that determine the possibility that the borrowing company would default. Banks analyze such information by primarily understanding the borrower's credibility.
Through this, they can formulate lending decisions to reduce the extent of their losses. Newer technology and programming languages have made it easier for banks to create credit risk analysis models.
Key Takeaways
- Borrowers, especially banks, use credit risk analysis models to quantify their credit risk using historical data and statistics.
- Corporate credit risk can be quantified and analyzed using the structural form and reduced-form models.
- Banks can assess a company's creditworthiness using their credit rating and an individual borrower using their credit score.
- To estimate the expected loss on default, the bank runs models that estimate the probability of default, exposure at default, and the loss-given default.
- Banks can make informed lending decisions and protect themselves from potential defaults by calculating the expected loss.
Understanding Credit Risk Analysis Modeling
Banks and financial institutions that provide company loans use credit risk analysis modeling. Risk modeling requires the quantification of historical data using statistics. They use these models to reduce their credit risk, whereby they are guaranteed to receive the principal and interest back.
The banks make their lending decision by evaluating the borrower's creditworthiness. If a borrower is deemed to have a higher likelihood of financial difficulty, the bank should refrain from extending credit to that particular company.
Judging the credit risk is crucial for a bank so that they do not experience any losses from a customer and can maintain their profitability. These banks constantly invest in newer technologies and systems that provide easy and quick models to quantify the borrower's credit risk.
Note
A part of their risk management strategy is quantifying and governing the credit risk influencing their financial performance. Credit risk can be easily identified and managed by employing such models.
Credit Risk Analysis models examine the borrower's performance for a period and evaluate the effect of adverse situations, such as a shift in the economy or revenue segments. Thus, the risk models provide a more enlightening approach to appraising credit risk.
Furthermore, as banks analyze the credit risk, they price the loan and levy interest according to the borrower's credit capacity. For companies with consistent income growth and a history of timely payments, the interest rate is usually lower compared to those with a bad credit repayment record.
Banks would prefer to invest in improving their systems, which assist in data collection and evaluating credit risk. Corporate credit risk can be quantified and analyzed using the structural form and reduced-form models.
- In a structural model, the firm's financial position is thoroughly scrutinized. Their balance sheet, which displays their assets, liabilities, and equities, is used to understand whether a company would default on a loan. The option pricing models, particularly the Black Scholes, are used to evaluate the probability of default.
- In a reduced-form model, default is considered an event that can occur and is not related to the firm's financial position. It does not consider the firm's assets and liabilities. Instead, it looks at default that could occur arbitrarily.
Types of Credit Risk Analysis Models
To model the credit risk, the lenders must run background checks to verify whether the borrower can repay the debt. This is done by evaluating a company's credit rating and an individual borrower's credit score.
Additionally, the other models assist in quantifying the probability of default, loss given default, and exposure to the borrower's bankruptcy. They help to evaluate the credit risk with a higher accuracy level.
The models for stress testing, machine learning, and portfolio credit risk models assist in creating data sets by analyzing realistic scenarios to estimate a relationship between the borrowers' characteristics and the default likelihood.
Credit Rating and Scoring Models
Credit ratings and scores are evaluations conducted by a third party to assess the borrower's creditworthiness. It is a widely used instrument that affects the capital decisions of the lender.
Risk-based pricing is a technique used by creditors that primarily evaluates the repayment ability of the borrower using credit history and ratings. Based on the ratings, they offer the borrower credit with an interest rate and loan term that suits them.
Note
Credit rating is given to businesses or corporations, whereas credit scores are given to individuals. Credit ratings can also be given to governments.
Some certified companies, including S&P Global, Fitch Ratings, and Moody's, provide credit ratings. These companies are popularly known as the Big Three. They independently assess the credit capacity of the borrowing company that issues debt as bonds.
Default risks are lower with a higher credit rating and vice versa. The credit ratings are assigned as a grade or letters, which depend on the type of company that is providing them.
In addition, they provide both short-term and long-term credit ratings. Short-term ratings are provided for a borrower's capacity to repay obligations that mature within a year.
Since debt is usually issued over a year, long-term ratings are more popular and relevant for credit risk modeling.
Among short-term ratings, P-1 (Prime - 1) is the lowest, and P-3 (Prime - 3 is the highest). Borrowers with a P-1 rating are most likely to repay their debts and have a superior credit repayment ability.
In contrast, long-term financing rating range from AAA to C. AAA-rated borrowers can fully pay their debts. On the other hand, C-grade borrowers are the lowest credit quality with very high credit risk.
Note
For more details regarding Moody's credit rating, click here.
Credit scores are assigned to individuals based on their repayment ability facilitated by their credit history. Companies that provide credit scores include Equifax, TransUnion, and Experian.
The credit score ranges from 300 (lowest credit score) to 850 (highest credit score). For example, the credit scores assigned by Experian range are as follows:
- 300-579 - Poor rating
- 580-669 - Fair rating
- 670-739 - Good rating
- 740-799 - Very good rating
- 800-850 - Exceptional rating
Probability of default (PD)
The probability of default (PD) measures the possibility that the borrower cannot repay their debt obligations resulting in a loan default. The expected loss can be estimated using the PD. The lender would need to know the borrower's credit rating and credit history to measure the default probability accurately.
Borrowers with a higher credit rating mostly have a lower probability of default, i.e., they would be able to service the loan throughout its term. On the other hand, borrowers with a lower credit rating will have a higher probability of default.
Additionally, the highly credit-worthy borrower will attract loans with a lower interest rate, whereas a lower credit-worthy borrower can only attract loans with a higher interest rate. The difference in the rate is due to the credit risk that the lender bears.
The Probability of Default can be estimated using the regression model, which relates the borrower's characteristics and default likelihoods.
PD = 1 / (1 + e^(-z))
Where,
- PD = Probability of Default
- e = 2.71828 (Eulers number - mathematical constant)
- z = relationship between the borrowers characteristics and default likelihoods.
Exposure at Default (EAD)
As the name suggests, the EAD measures the amount of loss a lender would be exposed to a contingent default. Banks predict this amount and base their lending decision on it.
As the borrower repays the loan, the overall probability of default reduces. The EAD is not a constant value as the default probability of the loan reduces as it approaches the final payment or the end of the loan term.
Note
The EAD can be estimated using the F-IRB or A-IRB Approaches. Both these approaches measure the credit risk as per the guidelines under the Basel II framework and set minimum capital requirements.
- F-IRB Approach: Banks internally estimate the probability of default. The other measures, including the Loss Given Default (LGD), are estimated by an independent third party or a regulator. Most importantly, the effect of collateral is not recognized.
- A-IRB Approach: The Advanced Internal Rating Based Approach allows banks to internally generate PD, LGD, and maturity factors. It provides a more flexible and relevant risk measure. Banks must publish risk exposures while adhering to regulatory guidelines.
The EAD could be equal to the loan amount if the bank has a reasonable expectation that the borrower cannot repay the whole loan; however, this is not so common. The EAD should be equal to the amount that is outstanding at the future point when the borrower could default.
Loss Given Default (LGD)
The LDG is the tool that measures the dollar amount of loss that would be incurred in the event of a default. It is an estimated percentage based on the EAD and other loan factors. The loan factors that affect the LGD include the collateral and the time to maturity or the loan term.
Collateral is an asset of the borrowing company pledged as a loan security. Loans, along with collateral, are more commonly called secured. They reduce the potential loss when the company defaults as the asset is transferred to the lender, who can sell the asset to recover some of the lost amounts.
To calculate the LGD, a bank needs to estimate the EAD and recovery rate. The recovery rate is the amount, usually a percentage, used to measure how much the bank would recover from the borrower in default.
LGD = EAD * (1 - recovery rate)
For example, a company approaches a bank to lend $800,000 with collateral as a security. The bank suspects that the lender will encounter financial difficulties and will default on the loan in the future.
It is estimated that it could happen when there is $100,000 outstanding. The recovery rate is 70%, which is high because of the collateral. Thus, the bank can estimate the LGD to be $30,000.
LGD = $100,000 * (1 - 0.7) = $30,000
Using all the above factors, a lender can compute the total expected loss that could be incurred after extending the loan. The expected loss is calculated using the formula below.
Expected Loss = PD * EAD * LGD
Other Types Of Credit Risk Analysis Models
In addition to these primary credit risk analysis models, analysts utilize stress testing, machine learning, and portfolio credit risk models.
- Stress testing models: These models evaluate the magnitude of credit risk when different assumptions are applied. It could be a scenario or sensitivity analysis.
- Machine learning models: These models create patterns from a dataset. These models can either be supervised (uses regression) or unsupervised. With the help of this, the lender can make predictions for the borrowers' credibility.
- Portfolio credit risk models: These models assess a bank's credit portfolio's overall credit risk. It estimates the probability of default by directly correlating the data with the underlying credit risk factors.
Credit Risk Analysis Factors
Various factors can affect the accuracy and effectiveness of credit risk analysis models. Below are some key factors that can impact credit risk analysis models.
Data Quality
The data set is considered good quality only when the information can assist decision-making. Generally, there are five characteristics to understand whether a data set is high quality. These are accuracy, completeness, timeliness, relevance, and reliability.
If the acquired data does not fit the following characteristics, there could be errors in the credit risk computation.
Economic Conditions
It is the economy's present state in a country or region. A country's economy is affected by multiple characteristics, especially interest rates, exchange rates, wages, labor, inflation, etc.
The economic stage - boom or recession - is a factor of uncertainty. The borrowers' credit risk is always affected by the economic condition. Banks need to steer themselves away from uncertainty related to the economy.
Uncertainty mainly refers to the characteristics that affect the economy. For example, an economy could be highly volatile once high inflation, low unemployment, and rising interest rates occur.
Industry-Specific Factors
These factors mainly relate to the industry that the borrower is employed. Every industry has different factors that would affect its credit risk, which must be incorporated while formulating the models.
For example, the automotive industry would be affected by its current trends, market share, sales, and expansion to Electric Vehicles (EVs).
Regulatory Environment
Regulations are the rules and guidelines an entity must adhere to while conducting business. The regulatory environment always impacts a business.
The magnitude of the impact depends on which industry the business is working under. Some highly regulated industries are - oil & gas, telecommunication, power generation, and transmission.
Borrower Characteristics
The borrower's characteristics are critical to evaluate before extending credit. More commonly, evaluating a borrower is done using the 5Cs. These include the character, capacity, capital, condition, and collateral.
Model Assumptions
The structural form of credit risk models mainly uses assumptions in its credit risk evaluation. The assumption is utilized for the interest rate. The interest rates are volatile in the short and long term.
Model Complexity
The model complexity refers to the number of parameters considered while computing credit risk. It may not always be possible to include every data in the model; however, the more data is included, the more complex the data set becomes.
Model Validation and Backtesting
Model validation is evaluating every aspect of the model to ensure its accuracy. Backtesting is evaluating whether the assumptions used in the model are accurate. It compares the forecasted results from the assumptions.
Market and Portfolio Segmentation
Portfolio segmentation, or customer segmentation, separates the portfolio into minor sections established on defined characteristics, mostly customers with similar risk characteristics. It is a risk management strategy.
Management Expertise and Judgment
The last factor that affects the credit risk models is the judgment made by the management concerning the loan. The management would use their skills and expertise to judge whether a borrower can repay the loan, thus managing its credit risk.
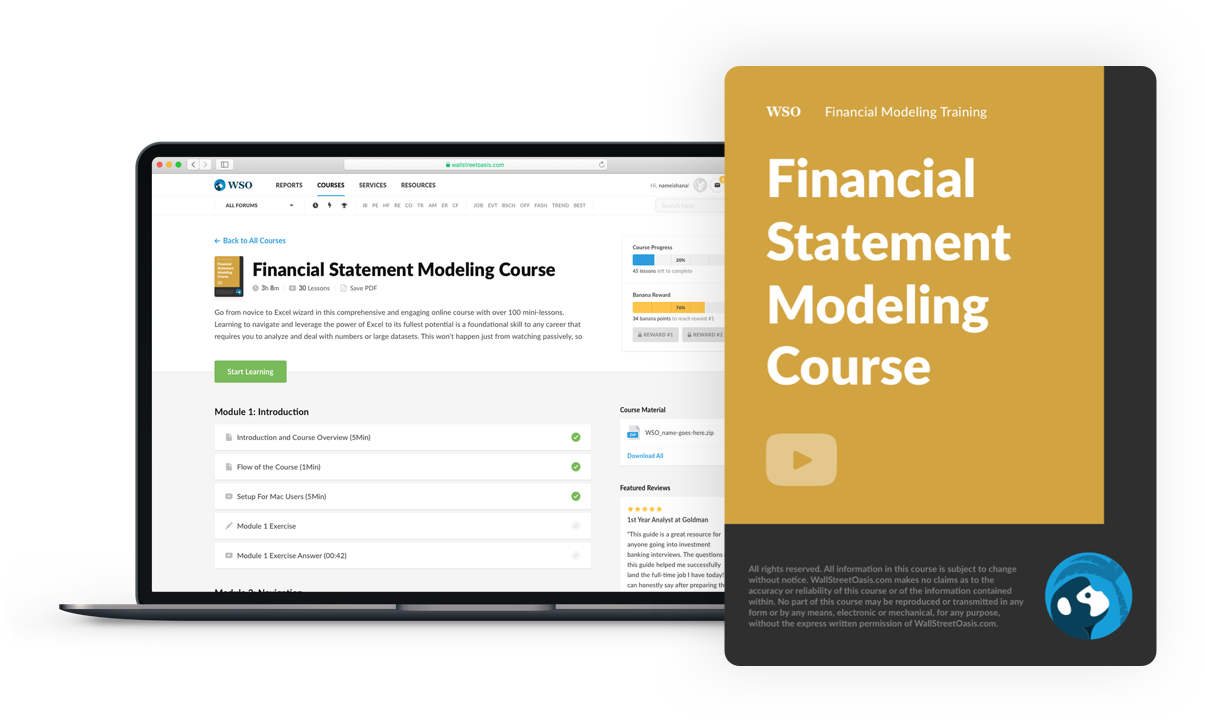
Everything You Need To Master Financial Statement Modeling
To Help You Thrive in the Most Prestigious Jobs on Wall Street.
or Want to Sign up with your social account?